Applying transaction risk rating systems
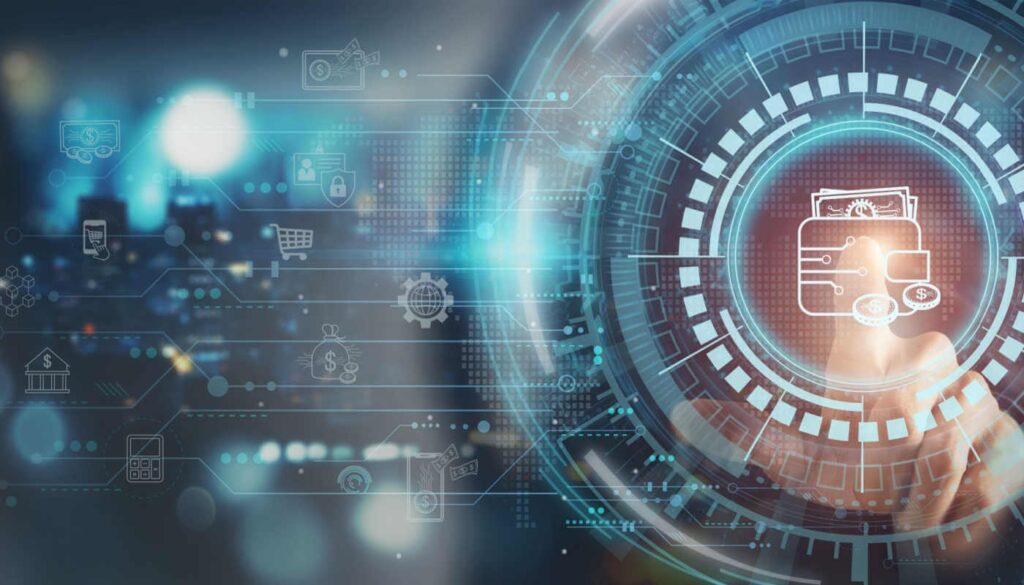
In financial services and financial technology, the growth in transactions via the Internet has resulted in an additional phase of complex fraudulent dangers, requiring the rapid implementation of modern safety precautions. Transaction risk score models, which use a variety of criteria, appear as an effective defense against these types of risks. The use of such models not only improves identifying fraud skills but also ensures compliance with law, especially when dealing with laws regarding money laundering. Know more payment risk scoring API
The Basis and Importance of Transaction Risk Score Systems
At the foundation of transaction risk score systems is a complex computational architecture that uses artificial intelligence and machine learning techniques to filter out and evaluate different purchase-related variables. These algorithms specialize in identifying transactions with a high risk, allowing banks to detect possible fraud or illicit financial operations. The basis of these frameworks is to evaluate several aspects, ranging from commercial facts like IP address and payment locations to more complex features like the transaction’s electronic trace which includes email and financial information. Integrating machine learning and artificial intelligence improves the model’s capacity to acquire knowledge from past information, constantly improving its estimated performance.
Data refinement and feature selection
Data refining and feature selection are critical elements in creating transaction risk score systems that accurately detect potential fraud. By carefully picking important variables and improving data, organizations can create algorithms that can identify existing fraud practices while also adapting to evolving hazards. This careful technique supports the creation of precise and legal designs, protecting the banking system from the combined risks of money laundering and fraudulent transactions.
Integrating subject knowledge and regulation guidelines
Successful choice of features and information refining are strongly dependent on industry knowledge and regulatory requirements. Compliance employees and fraud investigators are critical in determining which characteristics are most suggestive of risk, depending on the regulations and standard procedures. Furthermore, ongoing coordination with regulatory agencies guarantees that algorithms remain consistent with the most recent regulations on compliance and fraud prevention procedures.
One of the most difficult aspects of this procedure involves navigating the requirement for plenty of information with the possibility of excessive fitting, which occurs when the algorithm becomes too equipped with earlier information and loses its ability to predict new, previously unidentified information. To prevent this, cross-validating methods and frequent model assessments are required. Another problem is the constantly changing nature of money laundering and fraud strategies, which demands regular modifications to the set of capabilities and data improvement procedures to recognize new trends.
Managing and deploying the algorithms
The benefits of using risk-based scoring algorithms are numerous. They not only allow for the identification of fraud but also help to reduce errors in detection, which streamlines the transaction authorization procedure. They require frequent upgrades to stay up with emerging fraud strategies and may occasionally depend mostly on previous data, which can fail to catch new fraudulent activities. As a result, algorithms must be updated and improved frequently.